Today, approximately 6.5 million Americans are living with Alzheimer’s disease, a disorder that accounts for about 50-60% of all diagnosed neurodegenerative diseases, according to statisticscaptured by the Alzheimer’s Association. Because Alzheimer’s can only be definitively diagnosed via autopsy, these estimates may not be comprehensive.
Doctors need dependable methods to measure how abnormal changes in the brain take shape as dementia progresses. We may be one step closer to this future thanks to a new computational pipeline developed by Berkeley Lab’s Daniela Ushizima and UCSF Memory and Aging Center’s Lea Grinberg. The pipeline uses a variety of computer vision techniques, modern deep learning algorithms, and high-performance computing capabilities to process images of large-scale histological datasets – samples captured via autopsy and processed for microscopic examination – to generate quantitative 3D maps of abnormal protein deposits in whole human brains. Abnormal deposits of proteins, like tau inclusions, are hallmarks of Alzheimer’s disease and predictors of clinical decline.
On January 27, the 2023 Precision Medicine World Conference (PMWC) will recognize Ushizima and Grinberg with a Pioneer Award for constructing “a new and reliable technique for diagnosing Alzheimer’s disease and measuring the efficacy of experimental treatments.” The honor is typically given to “venerable individuals whose foresight and groundbreaking contributions to Precision Medicine propelled the movement in earlier years, allowing it to gain momentum to evolve into the standard of care it is becoming today.”
In addition to accepting PMWC’s Pioneer Award at the Santa Clara Convention Center, Ushizima will also lead the conference’s panel discussion on “Pattern Recognition and Content Quantification in Early-stage Disease Diagnosis.”
A Decade-Long Effort
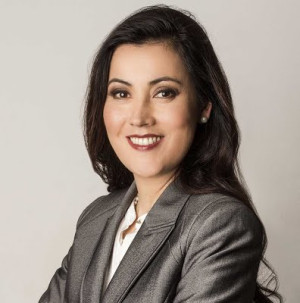
Daniela Ushizima
Over the past decade, applying multimodal imaging (combining data from more than one imaging technique) to precision medicine and advanced image analyses have helped transform health care. And in that time, researchers from Berkeley Lab and UCSF have been advancing this field even further by collaborating to create interactive multimodal visualizations of the brain using data collected from UCSF Memory and Aging Center (MAC).
In 2013, the team developed a computational tool with a unique user interface that allowed researchers to manipulate complex datasets. In one of their first demonstrations at the UCSF OME Precision Medicine Summit ten years ago, they unveiled a prototype built around a hand-gesture-controlled interface for brain data visualization developed in collaboration with Oblong Industries. The system offered a way to visualize data that could accelerate the pace of scientific discovery for neurodegenerative diseases.
In the years since that first demonstration, Grinberg’s team at UCSF MAC cultivated an extensive collection of brain images from dementia patients, collected via non-invasive in-vivo imaging (like PET scans) and immunohistochemistry, a laboratory method that uses antibodies to check for specific antigens (markers) in a sample of tissue, in postmortem human brains. The technique applied by Grinberg’s team checked for tau proteins.
According to the researchers, abnormal tau protein deposits form tangles inside the brain’s neurons; these deposits will cause cell death and brain shrinkage over time. The experts believe that understanding the position of these protein deposits in the brain and how they change over time is critical for gaining insights into how Alzheimer’s progresses. And this is where the Berkeley Lab and UCSF collaboration has been transformative.
Once the tau proteins had been identified, researchers would typically need to manually sift through all the labeled tissue samples and then try to quantify the number and size of the particles. They would then compare the immunohistochemistry data with positron emission tomography (PET) scans and try to calculate the co-occurrence of tau protein. PET scans give doctors an insight into the metabolic or biochemical function of tissues and organs. This test involves injecting a radioactive drug (tracer) into a patient’s bloodstream; the tracer will collect in areas with higher levels of metabolic or biochemical activity, which pinpoints the disease’s location often before it appears on other imaging tests like MRIs or CTs.
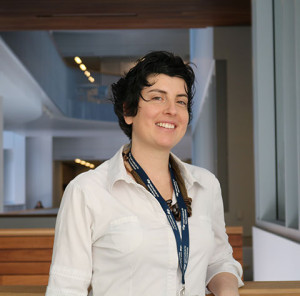
Lea Grinberg, UCSF
Manually identifying the association between tau proteins in immunohistochemistry and PET data is incredibly time-consuming and subjective; and has become a bottleneck in many laboratories. To overcome this challenge, Ushizima led an effort to determine a metadata and curation schema for these datasets and developed ways to annotate abnormalities consistently so that computer algorithms could learn to identify deposits of tau proteins. This foundation is what ultimately makes their new computational pipeline so successful.
Ushizima notes that a lot of work also went into identifying techniques and developing computational modules to align data collected from multiple instruments and methods – computed tomography (CT), magnetic resonance imaging (MRI), PET scans, autopsies, and more – into one multidimensional view of the brain. Using multimodal data allows for a finer-grain identification of anatomical regions associated with PET tracers and immunohistochemistry markers, which helps differentiate Alzheimer’s from other types of neuropathies and stage the disease, she explained.
“Aligning these different views of the brain is complex. Because these images are acquired via various in vivo methods, like CT and PET scans, they may have different spatial resolutions and deformations. In some cases, we were also overlaying pictures from brain slices captured from autopsies with MRI volumes,” said Ushizima. “To computationally align these brain views, we needed new registration modules. We also developed a new deep learning algorithm, called IHCNet, to measure microscopic specks corresponding to abnormal proteins in the brain.”
Ushizima notes that IHCNet is an image segmentation algorithm that uses a UNet “deep-learning” backbone to classify pixels belonging to abnormal tau deposits. This algorithm is at the core of the end-to-end pipeline that enables the creation of terabytes-large 3D tau inclusion density maps co-registered to MRI to facilitate the validation of PET tracers.
From Ushizima’s perspective, another great accomplishment of this collaboration between Berkeley Lab and UCSF has been the younger researchers that they’ve inspired to continue this work. Researchers like Silvia Miramontes, who began working on this project with Ushizima as part of the Science Undergraduate Laboratory Internship (SULI) program when she was an undergraduate student. Miramontes continued her work at Berkeley Lab while completing her four-year degree in applied math at UC Berkeley. This research also inspired her to pursue a Master’s in Information and Data Science from UC Berkeley while working at Berkeley Lab’s Center for Advanced Mathematics for Energy Research Applications (CAMERA). Now she’s working toward a Ph.D. in bioinformatics at UCSF.
“To me, this award is a testament to the power of team science and illustrates how the ‘data crunching’ we do can be transformative. Lea Grinberg is a neuroscientist, and I’m a computer scientist working in the business of computer vision. I investigate scientific experiments recorded as images that sometimes represent proteins in neuro data, sometimes they show calcium silicates in concrete, and sometimes they evidence battery quality from tomography scans. All of these investigations have a common mathematical data structure: matrices,” said Ushizima.
About Computing Sciences at Berkeley Lab
High performance computing plays a critical role in scientific discovery. Researchers increasingly rely on advances in computer science, mathematics, computational science, data science, and large-scale computing and networking to increase our understanding of ourselves, our planet, and our universe. Berkeley Lab’s Computing Sciences Area researches, develops, and deploys new foundations, tools, and technologies to meet these needs and to advance research across a broad range of scientific disciplines.
About Computing Sciences at Berkeley Lab
High performance computing plays a critical role in scientific discovery. Researchers increasingly rely on advances in computer science, mathematics, computational science, data science, and large-scale computing and networking to increase our understanding of ourselves, our planet, and our universe. Berkeley Lab's Computing Sciences Area researches, develops, and deploys new foundations, tools, and technologies to meet these needs and to advance research across a broad range of scientific disciplines.