This is a composite image of NGC 6357, a “cluster of clusters” located in our galaxy about 5,500 light years from Earth where radiation from hot, young stars energizes the cooler gas in the cloud that surrounds them, creating a cosmic dust “winter wonderland.” (Credits: X-ray by NASA/CXC/PSU/L.Townsley et al; Optical by UKIRT; Infrared by NASA/JPL-Caltech)
Tapping into the power of machine learning and artificial intelligence, a team led by Lawrence Berkeley National Laboratory (Berkeley Lab) scientists will host a series of competitions to spur future breakthroughs in high energy physics (HEP) and will work to build a supercomputer-scale platform where researchers can train and fine-tune their AI models.
The project is one of three team proposals – all connected to AI research in HEP – that recently received a share of $6.4 million in funding from the U.S. Department of Energy (DOE). The Berkeley Lab proposal’s title, “A Fair Universe: Unbiased Data Benchmark Ecosystem for Physics,” speaks both to scientific data management principles known as FAIR – short for “Findability, Accessibility, Interoperability, and Reusability” – and the creation of objective benchmarks for research.
The goal of the three-year effort is to develop an open-source platform with the help of the Perlmuttersupercomputer at the National Energy Research Scientific Computing Center (NERSC) at Berkeley Lab and then host benchmarks on that system to push the state of the art in AI for particle physics, said Wahid Bhimji, principal investigator on the project and group lead of the Data and Analytics Services Group at NERSC.
The collaboration comprises Berkeley Lab, the University of Washington, and ChaLearn, a nonprofit that organizes challenges to stimulate research machine learning. In addition to Bhimji, Fair Universe team members include Berkeley Lab’s Ben Nachman (Physics Division), Peter Nugent (Applied Mathematics and Computational Research Division), Paolo Calafiura (Scientific Data Division), and Steve Farrell (NERSC); Shih-Chieh Hsu (University of Washington); and Isabelle Guyon and David Rousseau (ChaLearn).
A Large-Scale AI Ecosystem
Machine learning benchmarks have pushed the state of the art in areas such as computer vision and language processing. The Fair Universe project aims to take AI to the next level for science.
“All these modern, deep-learning techniques that are coming out are being used in science, but there isn’t yet this transformative change that I think is possible,” Bhimji said. “For that to happen, scientists need these kinds of productive platforms for foundational AI that allows them to experiment and build general-purpose models and then apply them to different datasets.”
The new Fair Universe large-scale AI ecosystem will be used to share datasets, train and improve AI models, and host challenges and benchmarks.
“We’ve been thinking about different ways we can support and enable cutting-edge AI for science on NERSC supercomputers,” said Farrell, a machine learning engineer in NERSC’s Data and Analytics Services group. “Scientists want productive platforms that will enable them to more easily deploy their AI workloads on HPC systems. This project allows us to build such a capability.
There is also an opportunity for the collaborators to develop solutions that initially aid in hosting HEP competitions but can then be generalized across other science domains and different modes of research, he added. “Our FAIR data practices will additionally enable reproducibility in science, which is particularly important these days with the rapid progress in AI methods and applications.”
A key component of Fair Universe is the competitive challenges that will feature tasks of increasing difficulty based on novel datasets the project will provide. In recent years, members of the Fair Universe team have been involved in several machine learning challenges, Bhimji noted, including one around the Higgs boson particle discovered at CERN’s Large Hadron Collider (LHC).
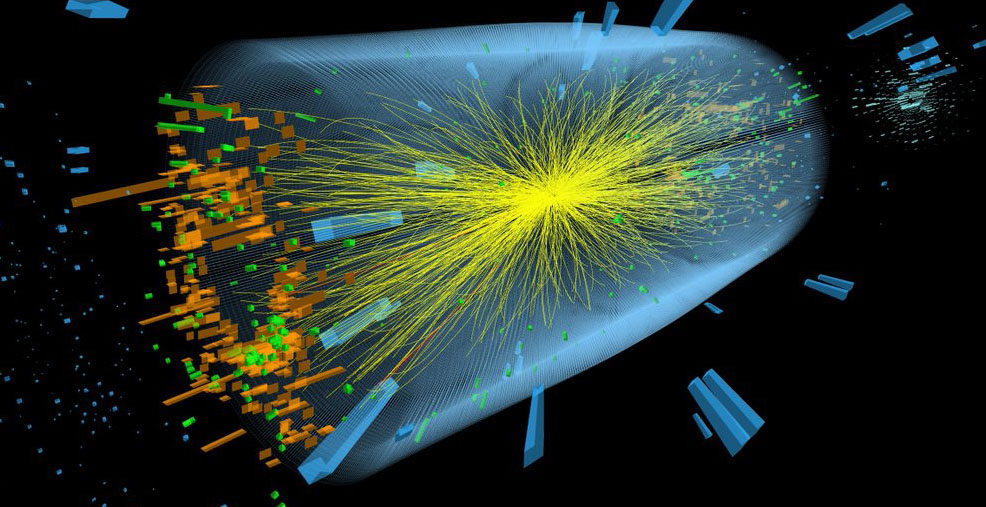
The Compact Muon Solenoid (CMS) experiment is one of two large, general-purpose particle physics detectors built on the Large Hadron Collider at CERN to search for new physics. (Credit: CERN)
“But now the kind of challenges in AI for particle physics and cosmology have moved on,” he said. “We need new techniques to account for that. And so the idea is to use this platform initially to run a challenge around uncertainty-aware machine learning techniques for particle physics.”
Accounting for Uncertainties
So what does “uncertainty-aware” mean? Imagine you’re looking to spot a cat in a picture using advanced deep learning techniques, but your deep learning cat-finder was trained on perfect images, while the real pictures you take have all kinds of flaws in the camera and it’s a 100 million super pixel camera – not unlike the LHC detectors with their 100 million channels and inevitable measurement errors. Now assume you not only want to find a cat in this picture, but you want to zero-in on a small tick on a cat that only occurs one in every billion cat pictures.
“So you want your algorithm to be both amazing at finding that very rare thing, but also to be able to tell you how confident it is that it found it, given the presence of all these differences between the slight imperfections with your camera and the perfect training data, which are the systematic uncertainties,” Bhimji said.
Developing systematic-uncertainty-aware AI models is critical to all areas of HEP, but a key challenge is the lack of datasets and benchmarks for research and development. As new methods are developed, it’s important to have common examples to compare performance. The Fair Universe team is eager to explore methods that can account for uncertainties because experimental facilities are becoming increasingly complex and measurements need to be more precise; in the case of the LHC, for example, detectors are the size of a building and have hundreds of millions of channels.
“Each of those can have uncertainties in the measurements they’re making,” Bhimji said. “So we really need machine learning techniques that are aware of the uncertainties in these detectors and can account for them.”
The hope is that new techniques could, for instance, help solve the vexing case of dark matter, one of the areas researchers at the LHC are trying to shed light on. But unless you have a method that accounts for uncertainties, you can’t find new hints of unknown particles that will hopefully explain dark matter, Bhimji explained.
Competitive Challenges
Looking ahead, the Fair Universe team will work to focus the attention of the HEP and AI communities on its competitive challenges, which will each take place over a period of two to three months. The code of the winning researchers will then be open-sourced and tasks will be publicly released and incorporated in a growing task benchmark to test novel methods that can be compared to the challenge-winning solutions. The plan is to have a series of competitions each year and then allow people to upload models and get scores back so they can test out their new models.
The trick will be to devise an initial challenge or problem that’s clear and universally accessible in order to draw a broad pool of competitors, Calafiura noted. Scientists trying to solve the problem should be able to just focus on the methods and not have to fight with preparing the data or understanding technicalities. But it’s a balancing act.
“This has to be done in a way that doesn’t dumb down the problem,” Calafiura said. “You want to simplify the problem but not oversimplify the problem.”
Bhimji sees the platform, and the project in general, benefiting an array of science and engineering communities.
“Ultimately the platform will be used for the whole range of NERSC science, including climate science, materials, and genomics,” he said.
About Computing Sciences at Berkeley Lab
High performance computing plays a critical role in scientific discovery. Researchers increasingly rely on advances in computer science, mathematics, computational science, data science, and large-scale computing and networking to increase our understanding of ourselves, our planet, and our universe. Berkeley Lab’s Computing Sciences Area researches, develops, and deploys new foundations, tools, and technologies to meet these needs and to advance research across a broad range of scientific disciplines.
About Computing Sciences at Berkeley Lab
High performance computing plays a critical role in scientific discovery. Researchers increasingly rely on advances in computer science, mathematics, computational science, data science, and large-scale computing and networking to increase our understanding of ourselves, our planet, and our universe. Berkeley Lab's Computing Sciences Area researches, develops, and deploys new foundations, tools, and technologies to meet these needs and to advance research across a broad range of scientific disciplines.