Computing Sciences Summer Students: 2020 Talks & Events
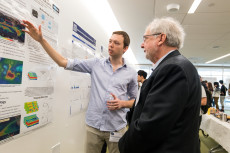
Computational Research Director David Brown listens as a summer researcher presents a poster detailing work done with Berkeley Lab mentors. (Credit: Margie Wylie for Berkeley Lab)
Summer Student Program Kickoff
Who: David Brown
When: June 02, 11 am - 12 pm
Where: Zoom (see calendar entry)
David Brown, Director of Computational Research Division (CRD), will kick off the CSSS program on Tuesday, June 2th at 11:00am with a special introduction of the virtual 2020 CSSS Program, and will describe the diverse areas of research currently undertaken by Berkeley Lab’s Computing Sciences. We look forward to your participation in this first virtual introductory talk of the 2020 Summer Student Program series.
David Brown has been Director of the Computational Research Division at Berkeley Lab since August 2011. His career with the U.S. Department of Energy (DOE) National Laboratories includes fourteen years at Los Alamos National Laboratory (LANL) and thirteen years at Lawrence Livermore National Laboratory (LLNL), where he was technical lead of several major research projects and held a number of line and program management positions. Dr. Brown's research expertise and interests lie in the development and analysis of algorithms for the solution of partial differential equations. He is particularly enthusiastic about promoting opportunities in computational science for young scientists from diverse backgrounds and is a founding member of the steering committee for the DOE Computational Graduate Fellowship Program. More recently, in collaboration with colleagues at Berkeley Lab and the Sustainable Horizons Institute, he helped create and promote the Sustainable Research Pathways program that brings faculty and students from diverse backgrounds for summer research experiences at Berkeley Lab. Dr. Brown earned his Ph.D. in Applied Mathematics from the California Institute of Technology in 1982. He also holds a B.S. in Physics and an M.S. in Geophysics from Stanford University.
Modeling Antarctic Ice with Adaptive Mesh Refinement
Who: Dan Martin
When: June 04, 11 am - 12 pm
Where: Zoom (see calendar entry)
Abstract: The response of the Antarctic Ice Sheet (AIS) remains the largest uncertainty in projections of sea level rise. The AIS (particularly in West Antarctica) is believed to be vulnerable to collapse driven by warm-water incursion under ice shelves, which causes a loss of buttressing, subsequent grounding-line retreat, and large (up to 4m) contributions to sea level rise. Understanding the response of the Earth's ice sheets to forcing from a changing climate has required the development of a new generation of next-generation ice sheet models which are much more accurate, scalable, and sophisticated than their predecessors. For example very fine (finer than 1km) spatial resolution is needed to resolve ice dynamics around shear margins and grounding lines (the point at which grounded ice begins to float). The LBL-developed BISICLES ice sheet model uses adaptive mesh refinement (AMR) to enable sufficiently-resolved modeling of full-continent Antarctic ice sheet response to climate forcing. This talk will discuss recent progress and challenges modeling the sometimes-dramatic response of the ice sheet to climate forcing using AMR.
Bio: Dan Martin is a computational scientist and group leader for the Applied Numerical Algorithms Group at Lawrence Berkeley National Laboratory. After earning his PhD in mechanical engineering from U.C. Berkeley, Dan joined ANAG and LBL as a post-doc in 1998. He has published in a broad range of application areas including projection methods for incompressible flow, adaptive methods for MHD, phase-field dynamics in materials, and Ice sheet modeling. His research involves development of algorithms and software for solving systems of PDEs using adaptive mesh refinement (AMR) finite volume schemes, high (4th)-order finite volume schemes for conservation laws on mapped meshes, and Chombo development and support. Current applications of interest are developing the BISICLES AMR ice sheet model as a part of the SCIDAC-funded ProSPect application partnership, and some development work related to the COGENT gyrokinetic modeling code, which is being developed in partnership with Lawrence Livermore National Laboratory as a part of the Edge Simulation Laboratory (ESL) collaboration.
Designing and Presenting a Science Poster
Who: Jonathan Carter
When: June 09, 11:00 am - 12:00 pm
Where: Zoom (see calendar entry)
Abstract: During the poster session on August 4th, members of our summer visitor program will get the opportunity to showcase the work and research they have been doing this summer. Perhaps some of you have presented posters before, perhaps not. This talk will cover the basics of poster presentation: designing an attractive format; how to present your information clearly; what to include and what not to include. Presenting a poster is different from writing a report or giving a presentation. This talk will cover the differences, and suggest ways to avoid common pitfalls and make poster sessions work more effectively for you.
Bio: Jonathan Carter is the Associate Laboratory Director for Computing Sciences at Lawrence Berkeley National Laboratory (Berkeley Lab). The Computing Sciences Area at Berkeley Lab encompasses the National Energy Research Scientific Computing Division (NERSC), the Scientific Networking Division (home to the Energy Sciences Network, ESnet) and the Computational Research Division.
NERSC: Scientific Discovery through Computation
Who: Rebecca Hartman-Baker
When: June 11, 11 am - 12 pm
Where: Zoom (see calendar entry)
Abstract: What is High-Performance Computing (and storage!), or HPC? Who uses it and why? We'll talk about these questions as well as what makes a Supercomputer so super and what's so big about scientific Big Data. Finally we'll discuss the challenges facing system designers and application scientists as we move into the exascale era of HPC.
Bio: Rebecca Hartman-Baker leads the User Engagement Group at NERSC, where she is responsible for engagement with the NERSC user community to increase user productivity via advocacy, support, training, and the provisioning of usable computing environments. She began her career at Oak Ridge National Laboratory, where she worked as a postdoc and then as a scientific computing liaison in the Oak Ridge Leadership Computing Facility. Before joining NERSC in 2015, she worked at the Pawsey Supercomputing Centre in Australia, where she coached two teams to the Student Cluster Competition at the annual Supercomputing conference, led the HPC training program for a time, and was in charge of the decision-making process for determining the architecture of the petascale supercomputer installed there in 2014. Rebecca earned a PhD in Computer Science from the University of Illinois at Urbana-Champaign.
Introduction to NERSC Resources
Who: Helen He
When: June 11, 2:00 pm - 4:00 pm
Where: Zoom (see calendar entry)
Abstract: This class will provide an informative overview to acquaint students with the basics of NERSC computational systems and its programming environment. Topics include: systems overview, connecting to NERSC, software environment, file systems and data management / transfer, and available data analytics software and services. More details on how to compile applications and run jobs on NERSC Cori will be presented including hands-on exercises. The class will also showcase various online resources that are available on NERSC web pages.
Bio: Helen is a High Performance Computing consultant of the User Engagement Group at NERSC. She has been the main point of contact among users, system people, and vendors, for the Cray XT4 (Franklin), XE6 (Hopper) systems, and XC40 (Cori) systems at NERSC in the past 10 years. Helen has worked on investigating how large scale scientific applications can be run effectively and efficiently on massively parallel supercomputers: design parallel algorithms, develop and implement computing technologies for science applications. She provides support for climate users and some of her experiences include software programming environment, parallel programming paradigms such as MPI and OpenMP, scientific applications porting and benchmarking, distributed components coupling libraries, and climate models.
Unitary Matrix Decompositions for Quantum Circuit Synthesis
Who: Roel Van Beeumen
When: June 16, 11 am - 12 pm
Where: Zoom (see calendar entry)
Abstract: On a quantum computer, a unitary transformation must be implemented by a quantum circuit. This circuit consists of a network of quantum gates, each performing a simple unitary transformation on one or a few qubits. The process of choosing and connecting these elementary quantum gates to achieve a unitary transformation of an n-qubit input is known as quantum circuit synthesis or quantum compiling. Mathematically, this amounts to decomposing a 2^n × 2^n unitary matrix into a product of simpler unitary matrices, where each of them is a sum of Kronecker products of simple 2 × 2 matrices and elementary unitary matrices. Because the decomposition of a unitary matrix in terms of elementary unitaries is not unique, multiple circuits can be generated for the same unitary transformation. However, to make the circuit efficient, additional requirements in terms of the total number of quantum gates, or circuit depth, and noise levels must be considered.
Bio: Roel Van Beeumen is a Research Scientist in the Computational Research Division at Berkeley Lab. His research interests range from numerical linear algebra and software for solving large-scale and high dimensional eigenvalue problems to quantum computing and quantum circuit synthesis. He earned his PhD in Engineering Science: Computer Science (2015) at KU Leuven in Belgium, from which he also holds Master degrees in Mathematical Engineering (2010) and in Archaeology (2011). Roel Van Beeumen is a recipient of the 2019 LDRD Early Career Award.
Crash Course in Supercomputing
Who: Rebecca Hartman-Baker
When: June 17, 10 am - 12 pm and 1 pm - 3 pm
Where: Zoom (see calendar entry)
Abstract: In this two-part course, students will learn to write parallel programs that can be run on a supercomputer. We begin by discussing the concepts of parallelization before introducing MPI and OpenMP, the two leading parallel programming libraries. Finally, the students will put together all the concepts from the class by programming, compiling, and running a parallel code on one of the NERSC supercomputers.
Bio: Rebecca Hartman-Baker leads the User Engagement Group at NERSC, where she is responsible for engagement with the NERSC user community to increase user productivity via advocacy, support, training, and the provisioning of usable computing environments. She began her career at Oak Ridge National Laboratory, where she worked as a postdoc and then as a scientific computing liaison in the Oak Ridge Leadership Computing Facility. Before joining NERSC in 2015, she worked at the Pawsey Supercomputing Centre in Australia, where she coached two teams to the Student Cluster Competition at the annual Supercomputing conference, led the HPC training program for a time, and was in charge of the decision-making process for determining the architecture of the petascale supercomputer installed there in 2014. Rebecca earned a PhD in Computer Science from the University of Illinois at Urbana-Champaign.
RECORDING (part 1)
RECORDING (part 2)
High Performance Computing For Cosmic Microwave Background Data Analysis
Who: Julian Borrill
When: June 18, 11 am - 12 pm
Where: Zoom (see calendar entry)
Abstract: The Cosmic Microwave Background (CMB) is the last echo of the Big Bang, and carries within it the imprint of the entire history of the Universe. Decoding this preposterously faint signal requires us to gather every-increasing volumes of data and reduce them on the most powerful high performance computing (HPC) resources available to us at any epoch. In this talk I will describe the challenge of CMB analysis in an evolving HPC landscape.
Bio: Julian Borrill is the Group Leader of the Computational Cosmology Center at LBNL. His work is focused on developing and deploying the high performance computing tools needed to simulate and analyse the huge data sets being gathered by current Cosmic Microwave Background (CMB) polarization experiments, and extending these to coming generations of both experiment and supercomputer. For the last 15 years he has also managed the CMB community and Planck-specific HPC resources at the DOE's National Energy Research Scientific Computing Center.
Rise of the Machines
Who: Prabhat
When: June 23, 11 am - 12 pm
Where: Zoom (see calendar entry)
Abstract: This talk will review progress in Artificial Intelligence (AI) and Deep Learning (DL) systems in recent decades. We will cover successful applications of DL in the commercial world. Closer to home, we will review NERSC’s efforts in deploying DL tools on HPC resources, and success stories across a range of scientific domains. We will touch upon the frontier of open research/production challenges and conjecture about the role of humans (vis-a-vis AI) in the future of scientific discovery.)
Bio: Prabhat leads the Data and Analytics Services team at NERSC. His current research interests include scientific data management, parallel I/O, high performance computing and scientific visualization. He is also interested in applied statistics, machine learning, computer graphics and computer vision. Prabhat completed his education from IIT Delhi, Brown University and UC Berkeley.
Surrogate Optimization for HPC Applications
Who: Juliane Mueller
When: June 25, 11 am - 12 pm
Where: Zoom (see calendar entry)
Abstract: High performance computing is crucial in many applications important to the Department of Energy for simulating complex physical phenomena, including climate sciences, high energy physics, and combustion research. These simulations usually contain parameters that determine how well the simulation represents reality. Optimizing these parameters is a computationally expensive task as it may take several minutes to hours to run the simulations with a given parameter set. Efficient optimization algorithms that do not rely on derivative information of the simulation objective function are needed. In this talk, we present an overview of surrogate model algorithms, which are commonly used to tackle these types of black-box expensive optimization problems. We discuss the importance of taking different problem characteristics into account when formulating the optimization problem and during the algorithm development and the potential impact on parallelizing these methods.
Bio: Juliane Mueller joined Berkeley Lab in 2014 as the Alvarez Fellow in Computing Sciences. She is now a research scientist in the Center for Computational Sciences and Engineering. Her research focuses on the development of efficient algorithms for solving computational expensive black-box optimization problems. Current application areas include high energy physics, optimal design, combustion, groundwater management, and quantum computing.
Efficient Scientific Data Management on Supercomputers
Who: Suren Byna
When: June 30, 11 am - 12 pm
Where: Zoom (see calendar entry)
Abstract: Science is driven by massive amounts of data. This talk will review data management techniques used on large-scale supercomputing systems. The topics include: Efficient strategies for storing and loading data to and from parallel file systems, querying data using array abstractions, and object storage for supercomputing systems.
Bio: Suren Byna is a Staff Scientist in the Scientific Data Management (SDM) Group in CRD @ LBNL. His research interests are in scalable scientific data management. More specifically, he works on optimizing parallel I/O and on developing systems for managing scientific data. He is the PI of the ECP funded ExaHDF5 project, and ASCR funded object-centric data management systems (Proactive Data Containers - PDC) and experimental and observational data management (EOD-HDF5) projects.
Neural Networks with Euclidean Symmetry for Physical Sciences
Who: Tess Smidt
When: July 02, 11 am - 12 pm
Where: Zoom (see calendar entry)
Abstract: Neural networks are built for specific data types and assumptions about those data types are built into the operations of the neural network. For example, full-connected layers assume vector inputs are made of independent components and 2D convolutional layers assume that the same features can occur anywhere in an image. In this talk, I show how to build neural networks for the data types of physical systems, geometry and geometric tensors, which transform predictably under rotation, translation, and inversion — the symmetries of 3D Euclidean space. This is traditionally a challenging representation to use with neural networks because coordinates are sensitive to 3D rotations and translations and there is no canonical orientation for physical systems. I present a general neural network architecture that naturally handles 3D geometry and operates on the scalar, vector, and tensor fields that characterize physical systems. Our networks are locally equivariant to 3D rotations and translations at every layer. In this talk, I describe how the network achieves these equivariances and demonstrate the capabilities of our network using simple tasks. I also present applications of Euclidean neural networks to quantum chemistry and geometry generation using our Euclidean equivariant learning framework, e3nn
Bio: Tess Smidt is the 2018 Alvarez Postdoctoral Fellow in Computing Sciences. Her current research interests include intelligent computational materials discovery and deep learning for atomic systems. She is currently designing algorithms that can propose new hypothetical atomic structures. Tess earned her PhD in physics from UC Berkeley in 2018 working with Professor Jeffrey B. Neaton. As a graduate student, she used quantum mechanical calculations to understand and systematically design the geometry and corresponding electronic properties of atomic systems. During her PhD, Tess spent a year as an intern on Google’s Accelerated Science Team where she developed a new type of convolutional neural network, called Tensor Field Networks, that can naturally handle 3D geometry and properties of physical systems. As an undergraduate at MIT, Tess engineered giant neutrino detectors in Professor Janet Conrad's group and created a permanent science-art installation on MIT's campus called the Cosmic Ray Chandeliers, which illuminate upon detecting cosmic-ray muons.
Challenges in Building Quantum Computers
Who: Anastasiia Butko
When: July 07, 11 am - 12 pm
Where: Zoom (see calendar entry)
Abstract: Building a quantum computer that can outperform classical super-computers is an extremely challenging task. It requires to address various research questions ranging from material science and electronics to create reliable qubit devices to classical architecture and software tools to support and control fundamentally different programming paradigm. A team of researches from different fields have united at the LBNL to address these question and challenges to bring quantum computing into the reality.
Bio: Anastasiia Butko, Ph.D. is a Research Scientist in the Computational Research Division at LBNL. Her research interests lie in the general area of computer architecture, with particular emphasis on high-performance computing, emerging and heterogeneous technologies, associated programming models and architectural simulation techniques. Her primary research projects address architectural challenges in adopting novel technologies to provide continuing performance scaling in the approaching Post-Moore’s Law era. Dr. Butko is a chief architect of the custom control hardware stack for the Advanced Quantum Tested at LBNL.
Simulating Supernovae with Supercomputers
Who: Donald Willcox
When: July 09, 11 am - 12 pm
Where: Zoom (see calendar entry)
Abstract: In this talk, I will present several kinds of astrophysics simulations showing how to model various astronomical processes on supercomputers, including Type Ia supernovae and core collapse supernovae. Type Ia supernovae are brilliant thermonuclear stellar explosions that provided the first direct evidence for the accelerating expansion of our universe. However, due to the rarity of Type Ia supernovae nearby, it is not well understood theoretically which stellar configurations lead to these explosions. In this talk, I will demonstrate that low Mach hydrodynamics coupled to nuclear reactions on adaptive meshes extends Type Ia supernova progenitor modeling to long time and small length scales in full-star three dimensional simulations. I will also show how extending these methods to include neutrino losses from weak nuclear reactions enables us to investigate systematic uncertainties previously out of reach for Type Ia supernovae models. I will also present my ongoing development work towards core collapse supernovae modeling as part of the ExaStar collaboration within the DOE Exascale Computing Project. Core collapse supernovae occur when a massive star's iron core collapses to form either a neutron star or black hole and present unique simulation challenges due to the intense neutrino radiation the neutron star emits. Along the way, I will point out how working at the intersection of astrophysics and computational science enables this exciting science.
Bio: Don Willcox is a postdoctoral researcher in the Center for Computational Sciences and Engineering (CCSE) in the Computational Research Division at Berkeley Lab. His research in computational astrophysics focuses on designing large scale hydrodynamics simulations of supernova explosions. He also develops efficient algorithms for ODE integration to model nuclear burning in various astrophysical processes and accelerates these algorithms for GPU-based supercomputers. Don completed his PhD in Physics at Stony Brook University in August 2018 working on thermonuclear supernovae modeling and the convective Urca process in white dwarf stars before joining Berkeley Lab.
Computing Beyond Moore’s Law
Who: John Shalf
When: July 14, 11 am - 12 pm
Where: Zoom (see calendar entry)
Abstract: Moore’s Law is a techno-economic model that has enabled the information technology industry to double the performance and functionality of digital electronics roughly every 2 years within a fixed cost, power and area. Advances in silicon lithography have enabled this exponential miniaturization of electronics, but, as transistors reach atomic scale and fabrication costs continue to rise, the classical technological driver that has underpinned Moore’s Law for 50 years is failing and is anticipated to flatten by 2025. This seminar provides an updated view of what a post-exascale system will look like and the challenges ahead, based on our most recent understanding of technology roadmaps. It also discusses the tapering of historical improvements, and how it affects options available to continue scaling of successors to the first exascale machine. Lastly, this seminar covers the many different opportunities and strategies available to continue computing performance improvements in the absence of historical technology drivers.
Bio: John Shalf is Department Head for Computer Science Lawrence Berkeley National Laboratory, and recently was deputy director of Hardware Technology for the DOE Exascale Computing Project. Shalf is a coauthor of over 80 publications in the field of parallel computing software and HPC technology, including three best papers and the widely cited report “The Landscape of Parallel Computing Research: A View from Berkeley” (with David Patterson and others). He also coauthored the 2008 “ExaScale Software Study: Software Challenges in Extreme Scale Systems,” which set the Defense Advanced Research Project Agency’s (DARPA’s) information technology research investment strategy. Prior to coming to Berkeley Laboratory, John worked at the National Center for Supercomputing Applications and the Max Planck Institute for Gravitation Physics/Albert Einstein Institute (AEI) where he was was co-creator of the Cactus Computational Toolkit.
Sparse Matrices Beyond Solvers: Graphs, Biology, and Machine Learning
Who: Aydın Buluç
When: July 16, 11 am - 12 pm
Where: Zoom (see calendar entry)
Abstract: Solving systems of linear equations have traditionally driven the research in sparse matrix computation for decades. Direct and iterative solvers, together with finite element computations, still account for the primary use case for sparse matrix data structures and algorithms. These sparse "solvers" often serve as the workhorse of many algorithms in spectral graph theory and traditional machine learning. In this talk, I will be highlighting some of the emerging use cases of sparse matrices outside the domain of solvers. These include graph computations outside the spectral realm, computational biology, and emerging techniques in machine learning. A recurring theme in all these novel use cases is the concept of a semiring on which the sparse matrix computations are carried out. By overloading scalar addition and multiplication operators of a semiring, we can attack a much richer set of computational problems using the same sparse data structures and algorithms. This approach has been formalized by the GraphBLAS effort. I will illustrate one example application from each problem domain, together with the most computationally demanding sparse matrix primitive required for its efficient execution. I will also briefly cover available software that implement these sparse matrix primitives efficiently on various architectures.
Bio: Aydın Buluç is a Staff Scientist and Principal Investigator at the Lawrence Berkeley National Laboratory (LBNL) and an Adjunct Assistant Professor of EECS at UC Berkeley. His research interests include parallel computing, combinatorial scientific computing, high performance graph analysis and machine learning, sparse matrix computations, and computational biology. Previously, he was a Luis W. Alvarez postdoctoral fellow at LBNL and a visiting scientist at the Simons Institute for the Theory of Computing. He received his PhD in Computer Science from the University of California, Santa Barbara in 2010 and his BS in Computer Science and Engineering from Sabanci University, Turkey in 2005. Dr. Buluç is a recipient of the DOE Early Career Award in 2013 and the IEEE TCSC Award for Excellence for Early Career Researchers in 2015. He is a founding associate editor of the ACM Transactions on Parallel Computing.
Advances on Graph-Based Machine Learning Algorithms for Image Analysis
Who: Talita Perciano
When: July 21, 11 am - 12 pm
Where: Zoom (see calendar entry)
Abstract: A large amount of research under DOE mission science relies on image-based data from experiments. The amount of data generated by user data facilities continues to rise due to increasingly productive state-of-the-art instruments. Experiments and simulations routinely generate datasets too large to analyze on a single machine. At the same time, the science questions being asked are increasing in complexity and sophistication. Although significant progress has been made, there is an opportunity to improve data analysis algorithms and software that are capable of extracting valuable and hidden information from scientific data efficiently. Such algorithms are essential to explore complex data, enabling accurate and deep understanding critical for decision-making.
Bio: Talita Perciano's work in the areas of image analysis, machine/deep learning, and high-performance computing is motivated by these science challenges. Her research focuses on mathematical foundations for new methods, on the implementation of scalable methods, and on platform-portability. Her goal is to develop powerful, mathematically-grounded, scalable algorithms that meet the requirements needed to analyze current and future scientific datasets acquired in user data facilities. She has been working closely with science stakeholders to help apply these methods and tools to their problems, developing work that impacts different scientific fields. In this seminar, Talita will present a few examples of current projects describing the problems addressed along with technical developments and scientific impacts.
RECORDING (part 1)
RECORDING (part 2)
From Simulations to Real-World: Building Deep Reinforcement Learning for Networks
Who: Mariam Kiran
When: July 23, 11 am - 12 pm
Where: Zoom (see calendar entry)
Abstract: Networks are the essential bloodline to science collaborations across the globe such as in high-energy physics, earth sciences, and genomics. We are exploring artificial intelligence and deep learning solutions to design and efficiently manage distributed network architectures to improve data transfers, guarantee high-throughput and improve traffic engineering
Bio: Mariam’s research focuses on learning and decentralized optimization of system architectures and algorithms for high performance computing, underlying networks and Cloud infrastructures. She has been exploring various platforms such as HPC grids, GPUs, Cloud and SDN-related technologies. Her work involves optimization of QoS, performance using parallelization algorithms and software engineering principles to solve complex data intensive problems such as large-scale complex simulations. Over the years, she has been working with biologists, economists, social scientists, building tools and performing optimization of architectures for multiple problems in their domain.
Towards a BES Light Source Wide Event-triggered Tomography Data Analysis Pipeline Using a Sustainable Software Stack
Who: Hari Krishnan
When: July 28, 11 am - 12 pm
Where: Zoom (see calendar entry)
Abstract: Scientific and technological advances in instrumentation at Basic Energy Sciences (BES) facilities are expected to result in petabytes of data per month, the consequence of these advancements have resulted in an increase in data hungry algorithms running at beamlines nationwide to study never before seen phenomena with ever greater richness in detail. Upgrades of ALS-U, APS-U, and LCLS-II are expected to come online resulting in exponential growth rate in data volume outstripping any foreseeable technological innovation in storage capacities making data retention an extremely challenging task. The current mode of siloed data storage methodologies and custom analysis pipelines driven is no longer a tenable option. Additionally, the decentralized process of past efforts have also resulted in data that is not well described, either semantically or structurally which makes writing anything but one-off implementations difficult. Data from the light sources is uniquely varied (many scientific techniques) and quickly changing (compared to say, climate satellites or telescopes where the structure of the raw data is fixed and rigorously specified). Moving from post-facto analysis (when all of the relevant data is available) to streaming analysis (when only some fraction of the data is available and you only see it once) requires changes to algorithms and mind-set. This talk will highlight development efforts as part of the work that is being undertaken for DOE light sources by the BES Data Pilot project and the Center for Advanced Mathematics for Energy Research Applications (CAMERA). Additionally, the talk will delve into the intricacies of deploying a real time event-driven nano-tomography processing pipeline with the aim to better understand the data pipeline and processing requirements needed for production environments. Tomography is a workhorse technique used at many scientific user facilities worldwide, and makes an ideal candidate in capturing the complexities of executing a multi-system distributed workflow in realtime.
Bio: Hari Krishnan has a Ph.D. in Computer Science and works for the visualization and graphics group as a computer systems engineer at Lawrence Berkeley National Laboratory. His research focuses on scientific visualization on HPC platforms and many-core architectures. He leads the development effort on several HPC related projects which include research on new visualization methods, optimizing scaling and performance on NERSC machines, working on data model optimized I/O libraries and enabling remote workflow services. As a member of The Center for Advanced Mathematics for Energy Research Applications (CAMERA), he supports the development of the software infrastructure, works on accelerating image analysis algorithms and reconstruction techniques. He is also an active developer of several major open source projects which include VisIt, NiCE, H5hut, and has developed plugins that support performant-centric scalable image filtering and analysis routines in Fiji/ImageJ.