Team ratchets up accuracy for identifying protein bits
March 30, 2011
Pacific Northwest National
Laboratory
William Cannon
William.Cannon@pnl.gov
Laboratory Web page
Pennsylvania State
University
Donald A. Bryant
dab14@psu.edu
University Web page
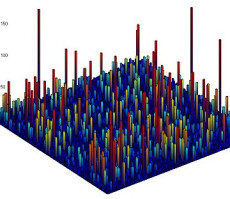
A team led by Pacific Northwest National Laboratory has come up with a new method to identify peptides, biologically significant protein fragments. Click image to enlarge and for more information. - See more at: http://ascr-discovery.science.doe.gov/feature/peptide1.shtml#sthash.aZGOkaey.dpuf
Anyone who has tried to match an unfamiliar bird’s features to its field guide portrait knows that reality rarely provides a perfect comparison to the ideal specimen.
Scientists have faced a similar problem when attempting to decode protein patterns found in living cells – a field known as proteomics. Using mass spectrometry, the technology of choice for protein identification, scientists try to match protein fragments, or peptides, against idealized patterns in peptide databases. These databases often provide a poor correspondence – the industry standard for positive peptide identification is usually a dismal 15 to 20 percent.
But using bioinformatics techniques, researchers at Pacific Northwest National Laboratory (PNNL) have developed a pattern-matching algorithm that improves the accuracy of peptide identification by between 50 and 150 percent, compared with standard approaches.
The key to the method, outlined this month in the online edition of the Journal of Proteome Research, was to deconstruct the pattern-matching problem using principles of statistical physics, which mathematically connects the behavior of individual atoms to large groups of molecules that can be observed and measured. The new method allows researchers to compare unknown peptide samples with both a peptide database of ideal samples and a library of experimental peptide samples.
The method is somewhat like having a field guide’s idealized bird plus numerous photographs of real birds in various poses to identify an unfamiliar bird among very similar unfamiliar birds. In the case of mass spectra, the PNNL scientists used a standard procedure for breaking apart proteins into component peptides, then separating the peptides from one another by their mass and charge. The resulting mass spectra are a series of lines of various heights signifying the amount and charge of each peptide fragment in a sample.
Current identification procedures take into account either idealized models of peptide spectra or highly detailed, realistic models analogous to photographs. By using both the presence of signature peaks and their characteristic heights in a manner inspired by chemical theory, the new algorithm represents both much more faithfully.
Using peak height enables researchers to go from black and white points of information to full color shapes that can be better matched to known peptides, says William Cannon, senior author of the Journal of Proteome Research paper and senior research scientist at PNNL.
“If you imagine the peak intensities as colors, now we are able to accurately match a much broader spectrum of colors,” Cannon says.
Solving the peptide identification problem is an urgent need because as soon as the first draft of the human genome sequence was complete in 2000, it quickly became apparent that medical advances based on genomic knowledge would require understanding how proteins carry out those genetic instructions. Deciphering medically meaningful protein patterns, often called protein biomarkers, has shown promise for diagnosing and treating disease and tracking infectious outbreaks, among other applications. But the proteome is many times more complex than the genome and changes in reaction to the environment. Being able to quickly identify new proteins made by the cell would hasten new medical treatments and help solve environmental challenges.
Teaming up to ID peptides
To solve the thorny peptide problem, Cannon revisited his roots in theoretical physics and chemistry to ask some basic questions about how molecules behave.
“We know from 100 years of theory that these probabilities, which are related to the energetics of the peptide, should be relatable to a count, based on statistical mechanics,” Cannon says. “We needed a mathematical score that leveraged the uniqueness of the mass spectra for each peptide.”
But developing an algorithm based on those first insights required the combined expertise of PNNL bioinformatician Mitchell Rawlins, computer scientist Douglas Baxter, microbiologist Stephen Callister, separations expert Mary Lipton and Pennsylvania State University collaborator Donald A. Bryant.
Cannon says the team’s approach is the first to “treat the spectra as a chemical process and ask how would you take into account peak height from a statistical physics standpoint.”
The goal was to come up with a statistical measure tailored to the unique characteristics of each peptide. Cannon knew from his training in physics that both thermodynamics and data analysis relied on determining probabilities.
Using methods inspired by thermodynamics, the new algorithm treats each peak mathematically as if it represents the count of the number of molecules present, then systematically includes peak height in identifying each unknown peptide. The final calculation gives the likelihood that an experimental peptide pattern matches a set of peaks of a known peptide.
The research team implemented the algorithm, called MSPolygraph, in both serial- and parallel-computing modes. For their experiments, they ran the code on supercomputers at the Environmental Molecular Sciences Laboratory, a national scientific user facility at PNNL, and the National Energy Research Scientific Computing Center at Lawrence Berkeley National Laboratory.
The final calculation gives the likelihood that an experimental peptide pattern matches a set of peaks of a known peptide. Investigators can then choose how strictly they want to assess the likelihoods. The more strict the assessment, the fewer peptides identified and the fewer false positive results. Conversely, the looser the assessment, the more peptides identified but the larger the error rate. The ability to identify a greater number of peptides at a fixed error rate makes the new technique more practical and useful than previous calculations.
The photosynthetic microbe test
To test their method, the group studied Synechococcus sp. PCC 7002, a type of marine cyanobacterium that can use the sun’s energy to convert carbon dioxide from the atmosphere into potential biofuels. Scientists are interested in this microbe because it can remove greenhouse gases from the atmosphere at normal atmospheric temperature and pressure. Little is known, however, about the proteins that accomplish what’s called photosynthetic carbon fixation.
With joint funding from DOE’s offices of Advanced Scientific Computing Research and Biological and Environmental Research, the scientists compared their new method of peptide identification with a widely used commercially available method. Using a set of 640,271 peptide spectra from the bacteria and high-performance computations, they correctly identified 125 percent more peptides using the new method, with a false positive rate of 5 percent.
What’s more, many of the newly identified peptides appeared to be involved in photosynthetic carbon dioxide fixation, the very process of most interest to the research team. For example, the number of peptides identifiable as participating in photosystem I, a key component in the conversion of sunlight to fuel, increased 160 percent. Whereas traditional peptide screening methods correctly identified three protein spectra involved in transporting CO2, the new hybrid search method found 951 such spectra, with a false discovery rate of 5 percent. The fact that the traditional method found only three spectra associated with the protein implied that the protein was not very abundant and hence not very important for growth in low CO2 conditions. In contrast, the new hybrid approach showed that this protein appears to be highly abundant at low CO2 and may therefore be important under these growth conditions.
“The ability to identify more peptides correctly allows one to far more accurately know the metabolic potential of cells,” says collaborator Don Bryant, Ernest C. Pollard professor of biotechnology at Penn State. “This information is crucial to building accurate metabolic models of Synechococcus to enhance biofuels production through metabolic engineering of the organism.”
Looking ahead, Cannon and his colleagues continue to refine the algorithm, and to incorporate MSPolygraph into cloud computing, where bioinformatics analysts could use it to interpret proteomics data.
The idea, Cannon says, is to provide a desktop interface for analysts to access supercomputing resources and visualize results by overlaying identified peptides, much like taking a scanned bird silhouette and overlaying it with potential species matches.
Short term, making peptide identification more visual and user friendly should speed matching and help cull out bad data. Down the road, Cannon sees a time when methods will have evolved to do predictive simulations.
About Computing Sciences at Berkeley Lab
High performance computing plays a critical role in scientific discovery. Researchers increasingly rely on advances in computer science, mathematics, computational science, data science, and large-scale computing and networking to increase our understanding of ourselves, our planet, and our universe. Berkeley Lab’s Computing Sciences Area researches, develops, and deploys new foundations, tools, and technologies to meet these needs and to advance research across a broad range of scientific disciplines.