NERSC Summer Students Take Deep Learning to the Next Level
September 1, 2020
Contact: cscomms@lbl.gov
Each summer, the National Energy Research Scientific Computing Center (NERSC) hosts several college-level students through the Computing Sciences Area’s Summer Student Program at Berkeley Lab. This year, many of the students focused on applying deep learning methods to an array of science applications, from weather and climate modeling to improving I/O scaling for GPUs.
“NERSC has been successful in attracting a diverse pool of interns every year, and we have seen a steady uptick in the number of interns who have expressed a desire to work on projects at the direct interface of deep learning and domain sciences,” said Prabhat, who leads the Data & Analytics Services (DAS) group at NERSC and has been instrumental in pioneering the application of deep learning to numerous science areas.
“Summertime is always intellectually stimulating because our talented interns are always pushing the boundaries of methods, software, and brand new application areas,” he added. “Over the years, we’ve seen internships result in posters, talks, and research papers at professional venues, and some projects have even morphed into award-winning Ph.D. dissertations.”
Here is a look at four students who spent the summer of 2020 doing research at NERSC – virtually, that is.
Ashesh Chattopadhyay
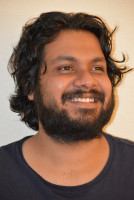
Ashesh Chattopadhyay
Ashesh Chattopadhyay is a third-year Ph.D. student at Rice University who obtained his undergraduate degree in mechanical engineering from the Indian Institute of Technology in Patna, India, and his masters in computational science from the University of Texas at El Paso.
His research experience is in the development of physics-informed deep learning algorithms in high-dimensional spatio-temporal turbulence, especially in climate dynamics and non-linear dynamical systems. At Berkeley Lab, his focus has been on developing deep learning algorithms that can be used to perform weather and climate modeling without any knowledge of the physical equations that govern the complex physics of these systems.
“This would open newer frontiers in terms of computationally efficient prediction of high-impact events such as extreme weather,” Chattopadhyay said.
His mentors at NERSC are Karthik Kashinath and Mustafa Mustafa, staff members in the DAS group who both have a growing body of expertise in this area.
“I've been familiar with their work in this field for a while now and have had the chance to interact with them at several conferences,” he said. “We have aligned interests in terms of research, so it only made sense to work with them over this summer on problems that we felt were important and interesting.”
For his Ph.D. research, Chattopadhyay is returning to his roots in mechanical engineering but with a focus on deep learning for high-dimensional systems such as turbulence and generally fluid dynamics.
“The fact is that deep learning algorithms were not designed for, or were not suitable for, applied science problems. But once adapted for these tasks, it can play a major role in rapidly facilitating development in the physical sciences,” he said. “A major challenge in problems such as climate/weather or generally fluid dynamics is the huge computational cost that is required for effective high-resolution simulations. Deep learning algorithms can play a major role in reducing these computational costs without compromising on the quality and accuracy of these simulations.”
Shuni Li
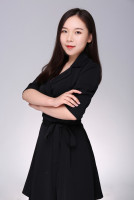
Shuni Li
Statistics is the core of machine learning and is the focus of Shuni Li’s Ph.D. work at U.C. Berkeley and her research at Berkeley Lab this summer.
“The project I have been working on over the summer has a lot in common with my Ph.D. research, which is about modeling and designing synthetic polymer sequences in materials science,” said Li, who has been working in the DAS group with mentor Steve Farrell. “They both try to model biological sequences and have the common goal of understanding what sequence compositions/structures allow certain sequences to function. When I saw in February that the intern project at NERSC - designing novel functional proteins using a generative model, variational autoencoder - was relevant to my own research interests , I immediately applied.”
Li’s undergrad work at Macalester College was in math and computer science, and she became interested in deep learning after watching a series of lectures on deep unsupervised learning by UC Berkeley’s Pieter Abbeel.
“When he talked about autoregressive models, latent models, generative adversarial networks, etc. and showed how they could be used to solve complex vision and language problems, I started to have this idea of combining deep learning with my own research,” she said. “And that’s when I started to explore relevant literature and build my own deep learning models to study synthetic biological sequences.”
Kevin Luna
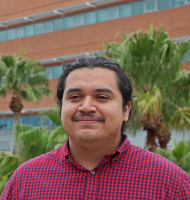
Kevin Luna
As a Ph.D. student in applied mathematics at the University of Arizona, the bulk of Luna’s training to this point has focused on traditional mathematical approaches to scientific problems. Along the way, he’s discovered that, while useful, these approaches have limits in their ability to produce answers to pressing scientific problems.
So, working with his mentor, Johannes Blaschke, and colleagues in the Data Science Engagement group, he’s leveraging deep learning methods to accelerate traditional partial differential equation-based simulations in real time. This work is now up for a Best Research Poster at SC20; Luna will give a presentation on this work at SC20 on Wed., Nov. 18, 11:07-11:30 am EST.
“The usual deep learning approach is to learn complex models capable of completely replacing direct calculations," Luna explained. "In practice, this comes at the cost of producing massive data sets (that are expensive to directly simulate in the first place). Our approach, which is an SC20 Best Research Poster Award finalist, instead seeks to use deep learning to reduce the cost of these expensive calculations with only limited data from a simulation."
Luna became interested in applying deep learning to science problems because of deep learning’s impressive performance and flexibility on a wide variety of scientific problems, he added. “However, as a mathematician at heart, rather than seeing deep learning as a means to replace traditional methods, I view it as a powerful complement to existing methods that we already have."
As a student with a budding research career, Luna added, “Berkeley Lab caught my interest because it provides a unique, supportive, and collaborative environment full of opportunities to learn from and work with world-class researchers on cutting-edge research problems.”
John Ravi
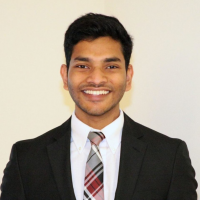
John Ravi
During his time at Berkeley Lab this summer, John Ravi has been making the most of his time by multi-tasking, working on different projects that aim to enhance I/O scaling for HPC, with a particular focus on improving GPU I/O for applications using HDF5 (version 5 of the Hierarchical Data Format for heterogeneous data).
“I am working on methods to improve the capability of applications to compute on larger data sizes in a shorter amount of time, in the hope that it would unlock more interesting findings,” he said. “I have been intrigued with what is possible with deep learning techniques ever since I first learned about them.”
A Ph.D. student at North Carolina State University – where he also earned his bachelors and masters degrees – Ravi’s background is in computer engineering and computer science. He was drawn to working at Berkeley Lab through his Ph.D. advisor, Michela Becchi, who had collaborated in the past with Suren Byna and Quincey Koziol, now his mentors at the Lab.
“I thought it would be a great opportunity to start a research collaboration that can extend past my summer internship,” Ravi said.
NERSC is turning out to be a go-to destination for students interested in working on deep learning for science, Prabhat added. “We’ve hosted domestic and international students; high school, masters, and Ph.D. students; and students pursuing degrees in statistics, applied mathematics, computer science, engineering, physics, and more,” he said. “We’d like to encourage students to watch out for our internship applications (typically advertised in January/February each year), and we hope to see you at Berkeley Lab very soon.”
About Computing Sciences at Berkeley Lab
High performance computing plays a critical role in scientific discovery. Researchers increasingly rely on advances in computer science, mathematics, computational science, data science, and large-scale computing and networking to increase our understanding of ourselves, our planet, and our universe. Berkeley Lab’s Computing Sciences Area researches, develops, and deploys new foundations, tools, and technologies to meet these needs and to advance research across a broad range of scientific disciplines.