HUNTRESS: A Step Forward for Computational Oncology Data Analysis
November 30, 2022
By Keri Troutman
Contact: cscomms@lbl.gov
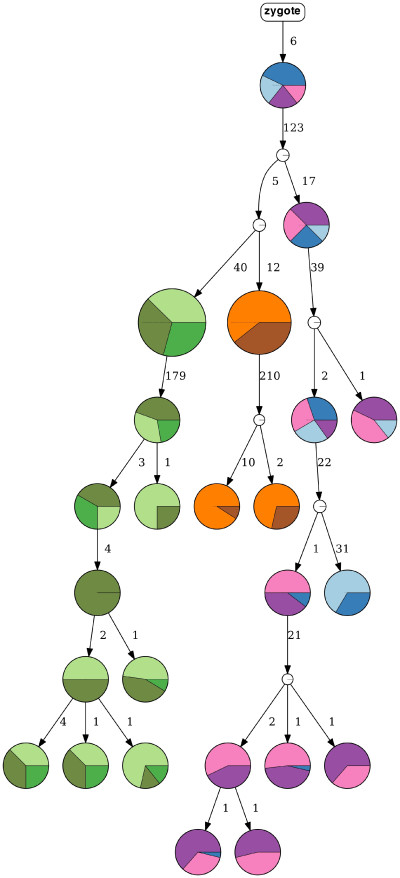
A tumor progression tree, inferred by HUNTRESS, represents nine clonal populations of high-grade serous ovarian cancer. (Credit: Can Kizilkale, Berkeley Lab)
The latest developments in computational oncology are giving medical researchers a glimpse into a future where they’ll be able to understand tumor progression via supercomputers and advanced mathematical algorithms. Toward this end, scientists from Lawrence Berkeley National Laboratory (Berkeley Lab) recently participated in a collaborative research project with the National Cancer Institute in which they developed HUNTRESS (Histogrammed UNion Tree REconStruction Scheme), a computational method that determines the true progression history of a tumor with high probability.
Tumors evolve from single cells, and tumor heterogeneity plays an important role in cancer progression. Identifying tumor heterogeneity is particularly important for understanding the gene expression patterns of individual cells.
This is where single-cell sequencing comes in. First introduced in 2009, this analytical method has revolutionized our understanding of the biological characteristics and dynamics of cancer lesions. It involves sequencing individual cells to obtain genomic, transcriptomic (an organism’s RNA transcripts), or multi-omics (genome, proteome, transcriptome, epigenome, and microbiome) information at the single-cell level – all datasets that have great potential for reconstructing the evolutionary history of tumors.
In the past decade, advances in single-cell sequencing technology have been followed by the design of various computational methods for inferring “trees” of tumor progression (tumor lineages are typically represented as tree structures). Some of these methods search for a solution directly in the tumor progression histories, while others perform the search among binary matrices that correspond to tumor progression trees.
Despite much progress, however, emerging larger-scale datasets remain a challenge for the available analytical capabilities. For example, available methods cannot handle datasets consisting of more than several hundreds of mutations and cells. These and related limitations led to the development of HUNTRESS, which employs mutational intratumor heterogeneity inference from noisy genotype matrices derived from single-cell sequencing data.
This approach allows HUNTRESS to achieve high accuracy while being much faster than state-of-the-art methods on all large datasets, according to a recent paper published in Nature Computational Sciences. On simulated and real tumor sequencing data, HUNTRESS was shown to be faster than available alternatives with comparable or better accuracy, noted lead author Can Kizilkale, a postdoctoral researcher in the Performance and Algorithms Research Group in Berkeley Lab’s Applied Mathematics and Computational Research (AMCR) division.
“This was a collaboration that leveraged each team’s strengths, our contribution being the development of the algorithm, its implementation, and work on the computation side, while our collaborators’ contribution was in providing the motivation on the cancer research side, designing experiments, and supplying and creating real and simulated data,” Kizilkale said.
Because of the Berkeley Lab team’s background in applied mathematics and optimization, he added, “we treated it as a matrix reconstruction problem.”
“The matrix is a challenge because it can be sparse: sequencing reads do not cover every position in the genomes of every cell,” said Aydin Buluç, a senior scientist in AMCR and co-author on the HUNTRESS paper. “Sometimes it is unknown because the genotyping is missing entries, and sometimes there are errors – false positives and false negatives. So the challenge for us was to come up with a reconstruction algorithm that gives not only accurate results but results in a short amount of time.”
Because cancer cells have heterogeneity, sometimes one cancer cell subpopulation is killed by therapy while others flourish, Buluç said. “So the therapy may kill a major subclone, but another possibly minor subclone may emerge and start dominating the tumor relapses as a result,” he explained. “Our paper is essentially a study of how distinct subpopulations emerge in tumor evolution and how they evade the immune system and therapeutic intervention.”
Huntress makes it possible to analyze datasets consisting of several thousands of cells in a matter of hours, while existing state-of-the art methods can not complete a similar task in several days. With all of its capabilities, HUNTRESS offers a timely advance in the resolution of intratumor heterogeneity and tumor progression history on datasets with increasing scale and complexity.
“With large matrices, it was almost impossible to use the methods that existed previous to our work because it was a matter of choosing between accuracy and speed,” said Kizilkale. “With HUNTRESS, as the number of samples in the matrix increases the accuracy of our algorithm also increases, so there’s a gain in two different directions: the more samples we have, the more accurate the method gets.”
Buluç and his group are now working on generalizing the technique developed for HUNTRESS to be applied in other research areas. Having access to resources at the National Energy Research Scientific Computing Center (NERSC) at Berkeley Lab will continue to be essential for this work, Buluç emphasized: “The more CPUs you have, the better this runs.”
NERSC is a U.S. Department of Energy Office of Science user facility.
About Computing Sciences at Berkeley Lab
High performance computing plays a critical role in scientific discovery. Researchers increasingly rely on advances in computer science, mathematics, computational science, data science, and large-scale computing and networking to increase our understanding of ourselves, our planet, and our universe. Berkeley Lab’s Computing Sciences Area researches, develops, and deploys new foundations, tools, and technologies to meet these needs and to advance research across a broad range of scientific disciplines.