Berkeley Lab Researchers Awarded ISQED’23 Best Paper
April 4, 2023
By Linda Vu
Contact: cscomms@lbl.gov
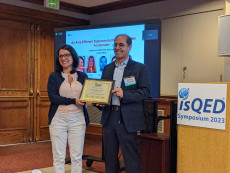
Berkeley Lab postdoctoral researcher Patricia Gonzalez-Gurrer is lead author of “An Area Efficient Superconducting Unary CNN Accelerator,” winner of the ISQED'23 Best Paper Award.
The International Symposium on Quality Electronic Design (ISQED’23) recognized researchers in Lawrence Berkeley National Laboratory’s (Berkeley Lab’s) Computer Architecture Group – Patricia Gonzalez-Guerrero, George Michelogiannakis, Kylie Huch, Nirmalendu Patra, Thom Popovici – with a Best Paper Award for “An Area Efficient Superconducting Unary CNN Accelerator.”
ISQED is the premier electronic design conference that bridges the gap between electronic and semiconductor ecosystems. It brings together experts from academia, industry, and government to highlight quality electronic design tools, integrated circuit technologies, semiconductor technology, packaging, assembly, and testing to achieve total design quality. This year’s conference will be held April 5-7 in San Francisco.
In their award-winning paper, the Berkeley Lab team uses an alternative computing paradigm called Unary Single Quantum Flux (U-SFQ) tailored to the technological advantages of superconductors. In a proof of concept, they explore a superconducting hardware accelerator for convolutional neural networks (CNNs) that are faster and smaller than state-of-the-art.
“Superconducting circuits are a very promising technology for the post-Moore era of computing. I believe that alternative computing paradigms like the one we propose could be the key to enabling practical superconducting computing for solving scientific problems,” said Gonzalez-Guerrero, a Berkeley Lab postdoctoral researcher and lead author on the paper.
Scientific Supercomputing in the Post-Moore Era
Over the past 30 years, advances in computer technology allowed processor performance and functionality to double every two years, a trend known as Moore’s Law. As computer chip manufacturing techniques reach the limits of the atomic scale, this era of predictable processor improvements is ending. In contrast, the computer processing demands of scientific applications continue to grow. So Berkeley Lab’s Computer Architecture Group is exploring alternatives to mainstream semiconductor technology, or complementary-metal-oxide-semiconductors (CMOS), to meet the increased processing demands.
One of the most promising post-Moore technologies is superconducting circuits, which are more energy efficient than CMOS semiconductors and can run at 50 to 70 GHz – significantly faster than contemporary CPUs with speeds ranging from 1.5 to about 5 GHz. But so far, superconducting prototypes have been limited to small-scale examples because of stringent area constraints (meaning that there isn’t enough space on the chip for computing components); and because researchers are applying CMOS-inspired voltage-level encoding techniques to these devices resulting in complex architectures that are at odds with the Single Quantum Flux (SFQ) pulses that superconductors use to carry information.
In their new paper, Gonzalez-Guerrero and her colleagues use an alternative computing paradigm called Unary SFQ (U-SFQ) tailored to the advantages of superconductors. U-SFQ uses two data representations: pulse streams and Race Logic (RL), and novel building blocks such as multipliers, adders, and memory cells, which use the natural properties of SFQ pulses to mitigate area constraints. Their proposed U-SFQ building blocks require up to 200 times fewer Josephson junctions (JJs) – the fundamental switching device in superconducting computing – than their SFQ binary counterparts, exposing an area-delay trade-off.
In a proof-of-concept, the team implemented a superconducting hardware accelerator for CNNs that is faster and smaller than state-of-the-art by mapping information to a unary format. CNNs are a network architecture for deep learning that learns directly from data; they are useful for finding patterns in images to recognize objects, classes, and categories. They can also effectively classify audio, time series, and signal data. Using their technique, the Berkeley Lab researchers achieved an area efficiency that gets superconducting technology closer to the practical implementation of realistic-size CNNs. With superconducting’s fast processing and energy efficiency, the proposed accelerator could be placed next to cryogenic sensors to achieve real-time processing.
“Winning this Best Paper Award makes me feel very encouraged,” said Gonzalez-Gurrero. “In research, you’re trying a lot of new things, and often you fail a lot more times than you succeed. This award encourages me to continue exploring new, high-risk, out-the-box concepts in my research.”
About Computing Sciences at Berkeley Lab
High performance computing plays a critical role in scientific discovery. Researchers increasingly rely on advances in computer science, mathematics, computational science, data science, and large-scale computing and networking to increase our understanding of ourselves, our planet, and our universe. Berkeley Lab’s Computing Sciences Area researches, develops, and deploys new foundations, tools, and technologies to meet these needs and to advance research across a broad range of scientific disciplines.