Autonomous Discovery: What’s Next in Data Collection for Experimental Research
CAMERA is sponsoring an April workshop on optimized data acquisition for instrument- and computer-based experiments
March 2, 2021
By Kathy Kincade
Contact: cscomms@lbl.gov
Marcus Noack of Berkeley Lab’s CAMERA Center will give a featured talk at SC21 on optimized data acquisition for instrument- and computer-based experiments. The virtual presentation will take place Tuesday, Nov. 16 at 11:30 a.m. CST. More information can be found on the DOE SC booth website.
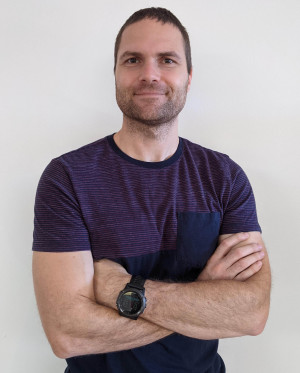
Marcus Noack is lead organizer for the April workshop on autonomous discovery.
Modern scientific instruments are acquiring data at ever-increasing rates, leading to an exponential increase in the size of data sets. Taking full advantage of these acquisition rates requires corresponding advancements in the speed and efficiency not just of data analytics algorithms but also of experimental control.
The goal of many experiments - both instrument-based and computer-based - is to gain knowledge about a material or process that is being studied. Experimentalists have a well-tested way to analyze a new material, taking samples of the material and measuring how it reacts to changes in its environment. For example, DOE Office of Science user facilities such as the Advanced Light Source and the Molecular Foundry at Lawrence Berkeley National Laboratory (Berkeley Lab) and the NSLS-II and the Center for Functional Nanomaterials at Brookhaven National Laboratory offer access to high-end-material characterization tools. But the associated experiments are often lengthy, and measurement time is precious.
“A standard approach for users at light sources is to manually and exhaustively scan through a sample,” said Marcus Noack, a research scientist in Berkeley Lab’s Center for Advanced Mathematics for Energy Research Applications (CAMERA). “But if you assume the data set is 3D or higher dimensional, at some point this exercise becomes infeasible. What is needed is something that can automatically suggest to the user what measurements should be performed next.”
Noack joined Berkeley Lab three years ago to bring mathematics into the design and optimization of experiments, with the ultimate goal of enabling autonomous experiments. One result of his efforts is gpCAM, a flexible algorithm that automatically selects measurements from an experiment and exploits Gaussian process regression to construct a surrogate model and an acquisition function based on the available experimental data. Mathematical function optimization is then used to explore an acquisition function to find a maximum and thereby suggest the location for the next measurement.
In this Q&A with Noack − lead organizer of a virtual workshop on autonomous discovery that will be held April 20-22 − he provides more details about this emerging data collection approach and the advantages it offers to multiple experimental and computational research areas.
In this brief video, Marcus Noak describes gpCAM, a tool developed at CAMERA for autonomous data acquisition. (Credit: Marcus Noak)
From your perspective as a mathematician, what is ‘autonomous discovery’?
Many experiments and simulations have what is called an underlying parameter space - that is, the outcome of the (computer) experiment depends on a combination of parameters. Often those parameters are plentiful, and it is impossible for a human to try them all or pick them by intuition. This is where autonomous discovery comes into play. Given a few results produced with different combinations of the parameters, a computer can look at the problem and autonomously steer what should happen next, either based on pure uncertainty computations or on some objective.
How does it differ from machine learning?
Autonomous discovery uses many techniques that are also part of the field of machine learning - and, more specifically, active learning. Autonomous discovery is based on the idea of function approximations through data, which also serves as a foundation for most machine learning techniques.
What advantages does autonomous discovery bring to scientific research?
The parameter spaces underlying today's experiments are vast and high-dimensional, and amazing science can hide anywhere within them. The smarter the algorithm, the more likely it is that a region can be identified where new science might be discovered. This can revolutionize quite literally every field in science and engineering. Not only can we find more, but we can also find it faster with the right algorithms, saving experimentalists a lot of time and effort.
Are there particular areas of scientific research where it is most relevant?
The techniques can be applied to almost every field in experimental sciences, and even to many in computational simulations, where a vast combination of input parameters and output results can exploit these ideas. Whenever some data is available and the question is what to do next, autonomous discovery can help.
What prompted the development of gpCAM?
The motivation to develop gpCAM came from beamline experiments. When I started, those experiments were largely steered by intuition and brute-force automated approaches, which can waste financial, human, and computer resources. We needed a very general, flexible, and easy-to-use algorithm that can assist practitioners with their experimental designs.
Has gpCAM’s application now expanded into other research areas?
Yes. In addition to beamline science, gpCAM is now used in North America and Europe for spectroscopy, microscopy, neutron scattering, computational simulations, and other specific applications.
What are the focus and goals of the April workshop, and who are you encouraging to attend?
The goal of the workshop is to create a community around autonomous discovery. It is such a new field and, for many, more of a myth than reality. We want to change this. One particular emphasis of the workshop is to welcome newcomers and early career researchers from a variety of scientific disciplines and introduce them to the methodology and applications.
About Computing Sciences at Berkeley Lab
High performance computing plays a critical role in scientific discovery. Researchers increasingly rely on advances in computer science, mathematics, computational science, data science, and large-scale computing and networking to increase our understanding of ourselves, our planet, and our universe. Berkeley Lab’s Computing Sciences Area researches, develops, and deploys new foundations, tools, and technologies to meet these needs and to advance research across a broad range of scientific disciplines.